You signed in with another tab or window. Reload to refresh your session.You signed out in another tab or window. Reload to refresh your session.You switched accounts on another tab or window. Reload to refresh your session.Dismiss alert
I'm using it to make some chaotic learning with thousands of model, and a greedy fitness function. the parallelization is realy efficient in my case.
I have found some problems with multithreading using keras models.
To reproduce the problem, i use this regression sample : https://pygad.readthedocs.io/en/latest/README_pygad_kerasga_ReadTheDocs.html#example-1-regression-example
I only reduce the num_generations to 100.
Steps to reproduce :
I run a few times the sample,
then, i enable the parallel processing on 8 threads :
then, run again a few times :
sometimes, i see in logs a fitness lower than the n-1 generation, example :
I printed all solutions used in each epoch, and i saw thats solutions are most of time the same, so the parallel_processing seems to break the generation of the next population in the most of cases.
Thanks!
EDIT :
In addition i tried to reproduce the same problem with this classification problem sample ,
Adding the multiprocessing support cause the same problem.
The text was updated successfully, but these errors were encountered:
Hi,
I realy appreciate your works on PyGAD!
I'm using it to make some chaotic learning with thousands of model, and a greedy fitness function. the parallelization is realy efficient in my case.
I have found some problems with multithreading using keras models.
To reproduce the problem, i use this regression sample :
https://pygad.readthedocs.io/en/latest/README_pygad_kerasga_ReadTheDocs.html#example-1-regression-example
I only reduce the num_generations to 100.
Steps to reproduce :
I run a few times the sample,
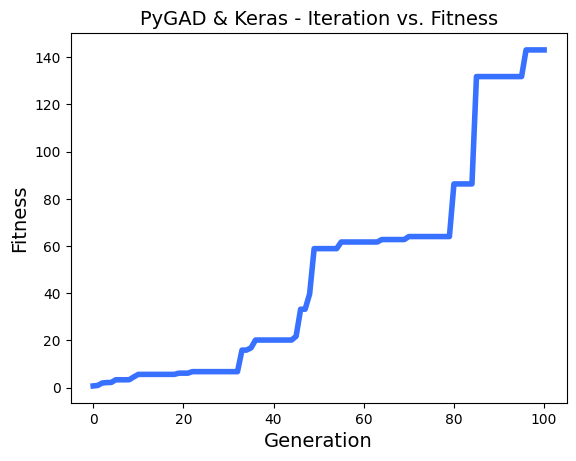
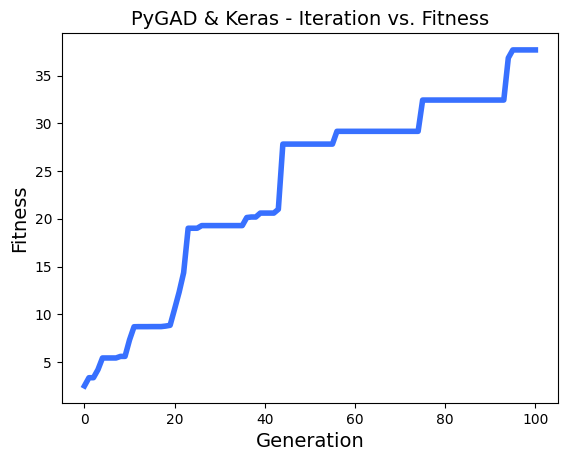
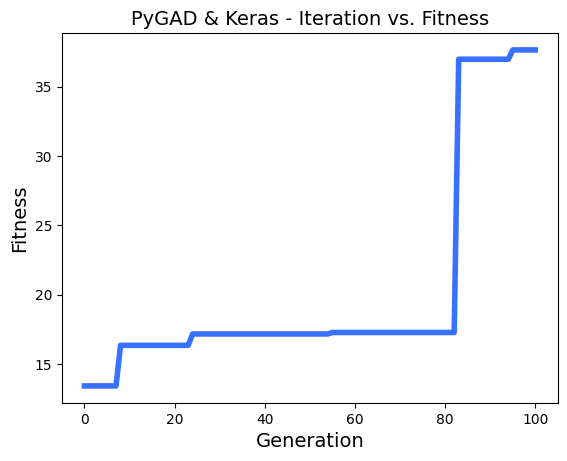
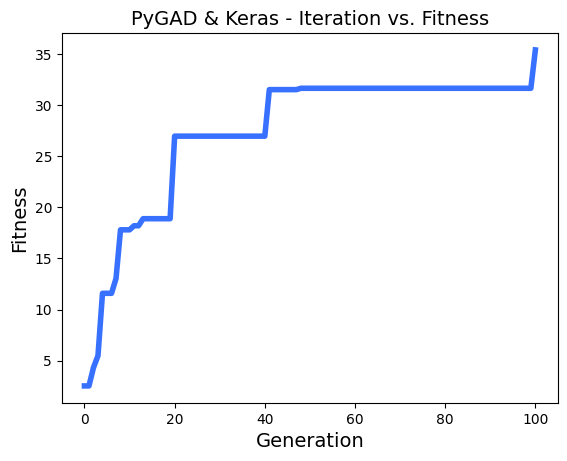
Thanks!
EDIT :
In addition i tried to reproduce the same problem with this classification problem sample ,
Adding the multiprocessing support cause the same problem.
The text was updated successfully, but these errors were encountered: